Deep Sports Pose
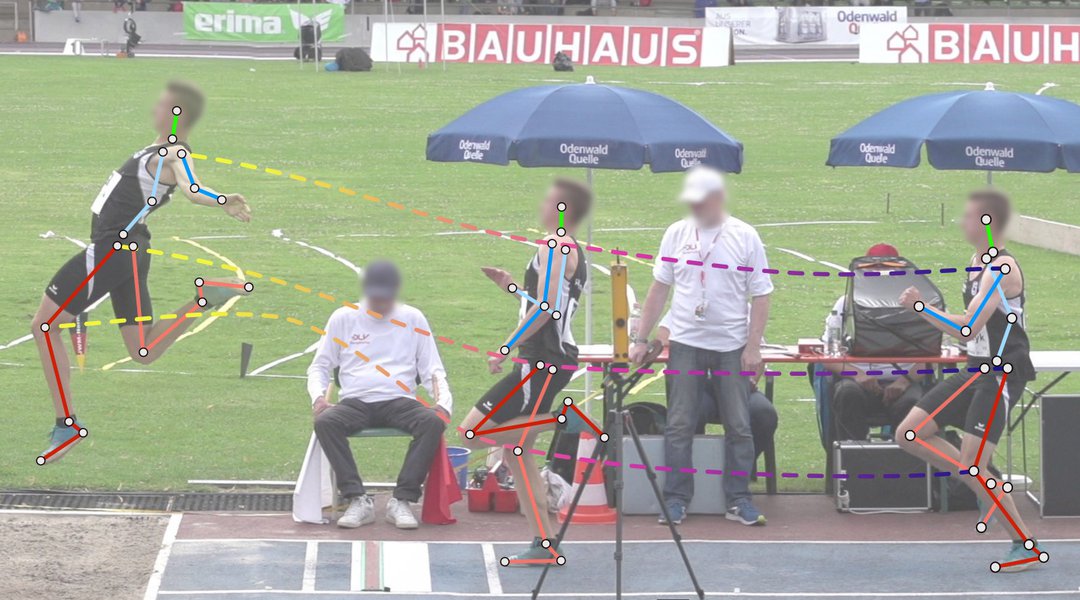
Video recordings of athletes are an important tool in many sport types, including swimming and long/triple jump, to evaluate performance and assess possible improvements. For a quantitative evaluation the video material often has to be annotated manually, leading to a vast workload overhead. This limits such an analysis to top-tier athletes only. In this joint project with the Olympic Training Centers (OSPs) Hamburg/Schleswig-Holstein and Hessen we research deep neural network based human pose estimation and video event detection that can be applied to various sport types and environments. We evaluate our research using the very different examples of start phases in swimming and long/triple jump recordings. Our main focus lies on time-continuous predictions and the fusion of multiple synchronous camera streams. The goal of the project is to provide a reliable and automatic pose and event detection system that makes quantitative performance evaluation accessible to more athletes more frequently.
This joint project is funded by the Federal Institute for Sports Science (Bundesinstitut für Sportwissenschaft, BISp) based on a resolution of the German Bundestag, starting January 2017.
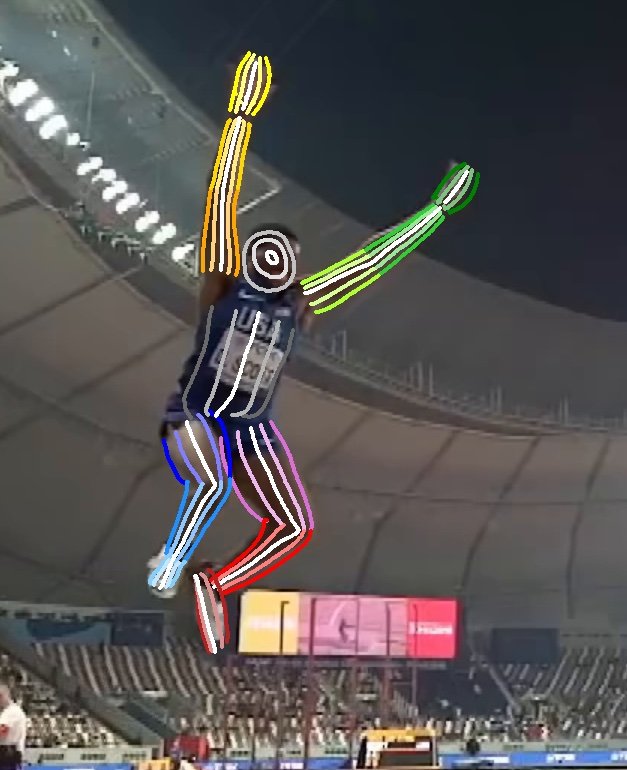
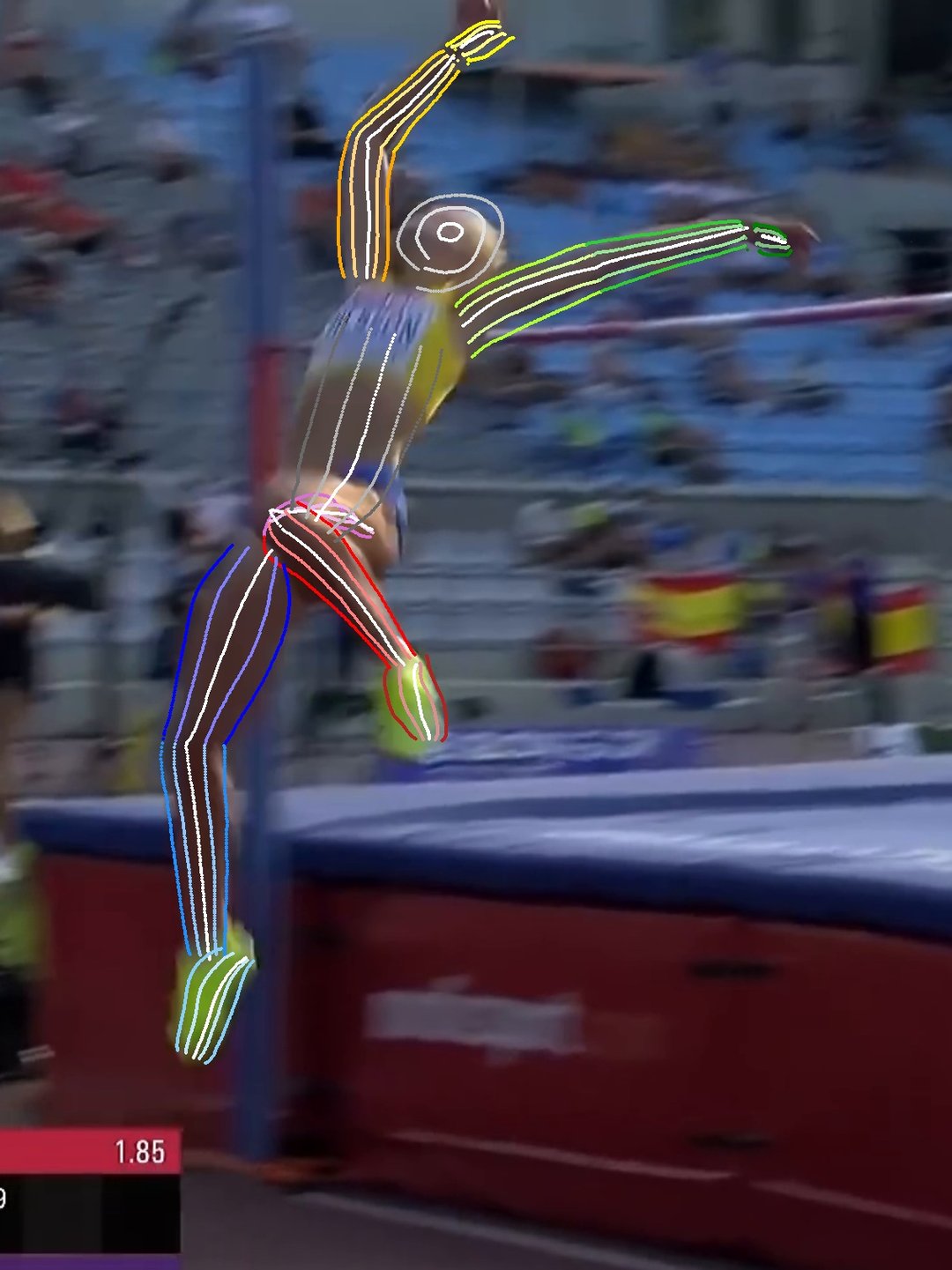
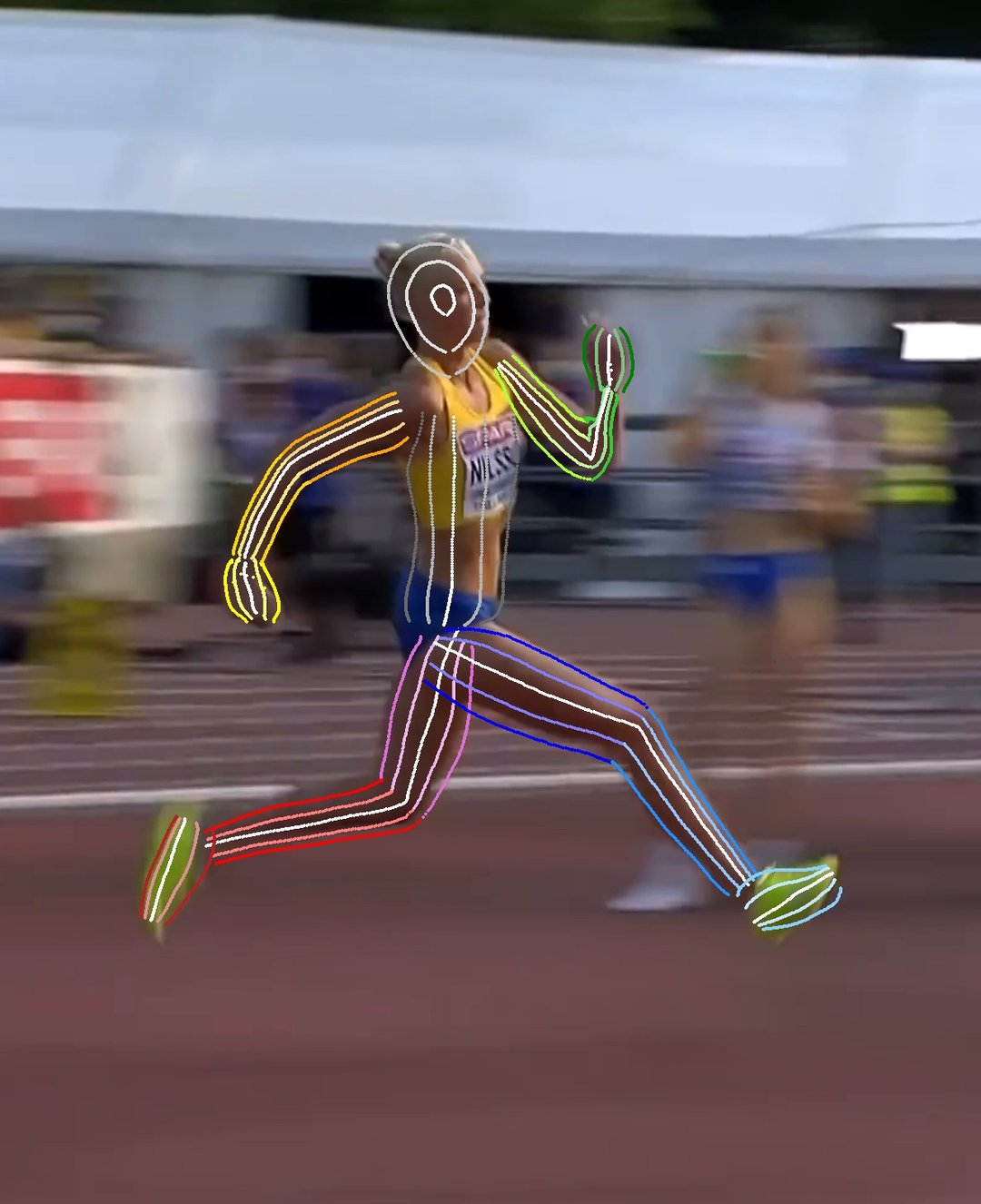
We further enhance our system to detect more than the hand-annotated keypoints. We train a VisionTransformer based model to detect arbitrary keypoints, meaning any keypoint that is located on the body of the athletes. This training is based on automatically generated segmentation masks which are often imprecise or incorrect, but our training process is able to cope with this challenge. We further improve the model to predict these points correctly on bent limbs (knees and elbows).
This joint project was funded by the Federal Institute for Sports Science (Bundesinstitut für Sportwissenschaft, BISp) based on a resolution of the German Bundestag.
For more information please contact Katja Ludwig.
References:
- Katja Ludwig, Julian Lorenz, Robin Schön, Rainer Lienhart.
All keypoints you need: detecting arbitrary keypoints on the body of triple, high, and long jump athletes.
2023 IEEE/CVF International Conference on Computer Vision and Pattern Recognition Workshops (CVPRW), June 17 2023 to June 24 2023, Vancouver, BC, Canada. - Katja Ludwig, Daniel Kienzle, Rainer Lienhart.
Recognition of freely selected keypoints on human limbs.
IEEE/CVF International Conference on Computer Vision and Pattern Recognition Workshops (CVPRW), New Orleans, LA, USA, 19-24 June 2022. - Moritz Einfalt, Rainer Lienhart.
Decoupling Video and Human Motion: Towards Practical Event Detection in Athlete Recordings.
The IEEE Conference on Computer Vision and Pattern Recognition (CVPR) Workshops 2020. Seattle, WA, USA, June 2020. [ arXiv CVF PDF] - Moritz Einfalt, Charles Dampeyrou, Dan Zecha, Rainer Lienhart.
Frame-level Event Detection in Athletics Videos with Pose-based Convolutional Sequence Networks.
Second International ACM Workshop on Multimodal Content Analysis in Sports (ACM MMSports'19), part of ACM Multimedia 2019. Nice, France, October 2018. [ ACM DL PDF] - Rainer Lienhart, Moritz Einfalt, Dan Zecha.
Mining Automatically Estimated Poses from Video Recordings of Top Athletes.
IJCSS, December 2018. [ IJCSS PDF] - Moritz Einfalt, Dan Zecha, Rainer Lienhart.
Activity-conditioned continuous human pose estimation for performance analysis of athletes using the example of swimming.
IEEE Winter Conference on Applications of Computer Vision 2018 (WACV18), Lake Tahoe, NV, USA, March 2018. [ arXiv IEEE PDF]
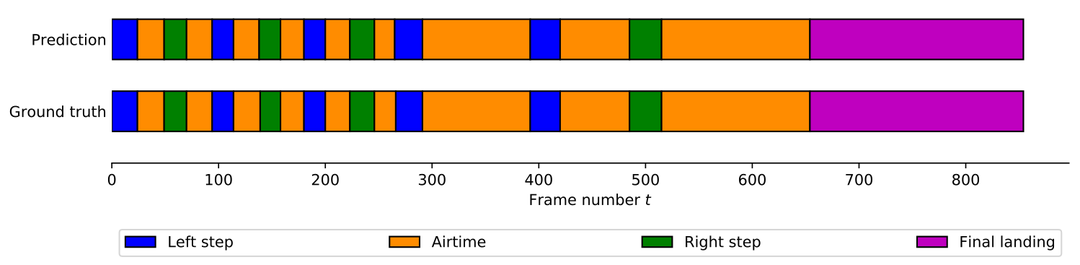
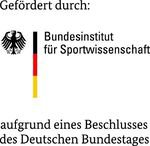