Real-time capable process modelling in production technology
Our research activities focus on scrap reduction and holistic process optimisation to reduce the use of resources in production processes.
Numerical simulations exist for many production processes, describing the physical processes on the basis of partial differential equations. These differential equations are typically solved using numerical methods such as the finite element method, and a solution is approximated based on the boundary conditions (process parameters, tooling, etc.). Finding these solutions is often very time-consuming, so the simulations are suitable for individual calculations but not for inverse solving of process problems (what parameters do I have to choose in the process to get a certain result?). The desire for inverse optimisation of process parameters inevitably leads to fast computational process models that are differentiable if possible. This enables the use of efficient optimisation algorithms from the field of data science.
The aim is to investigate how such fast surrogate models can be efficiently created and used for inverse process optimisation and real-time process control. A particular focus will be on combining data-based methods with engineering knowledge based on differential equations. Data-based methods have the potential to learn from experimental data effects such as friction and complex mechanical contacts, which are traditionally difficult to model using numerical methods. To minimise the costly generation of consistent training data, existing engineering knowledge will be integrated into the training (physically informed machine learning).
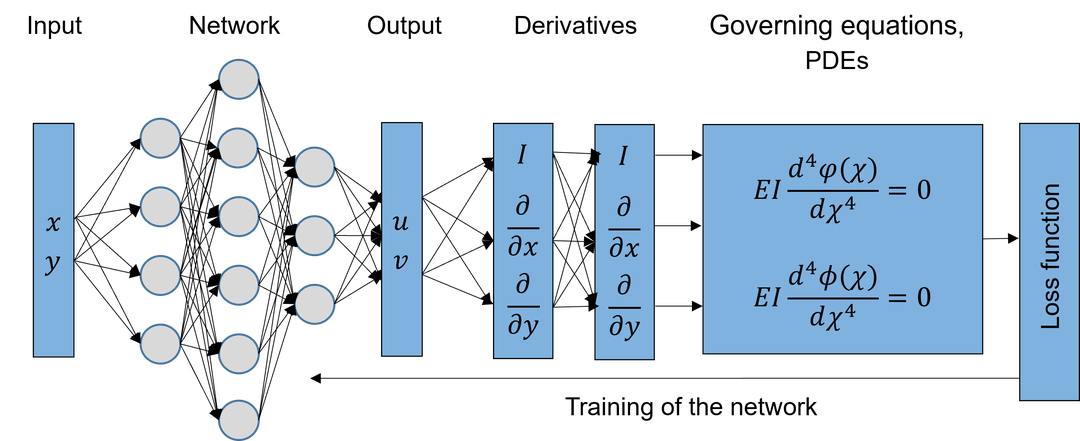
To improve production processes in the long term with this research strategy, the strategic objectives are systematically broken down into sub-aspects and developed sequentially from basic research to application-oriented research. We are currently actively researching the following processes:
- We are working on geometry control and optimisation in free-form bending.
- We study computational optimisation of the high-pressure die-casting process chain.