Projekte
ADELeS
Assistenzsystem zur KI-unterstützten Detektion und Behebung von Qualitätsabweichungen und Fehlern in Produktionsprozessen
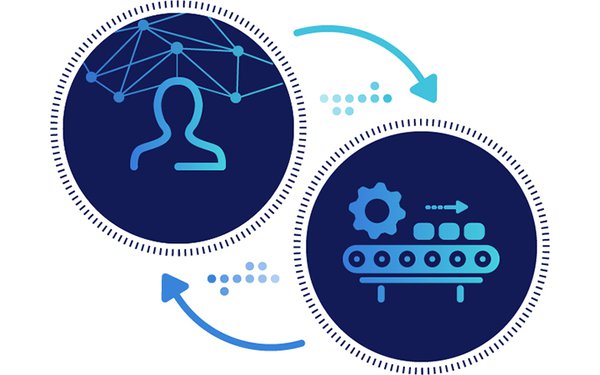
AICUT
Automatisierte Erkennung von Prozessstörungen und Qualitätsschwankungen bei der spanenden Fertigung mittels maschinellen Lernens
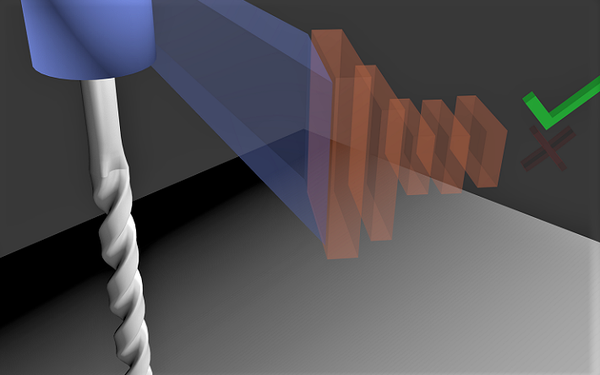
Kiko.BD
Entwicklung KI-gestützter Regelungsverfahren sowie eines digitalen Zwillings zum Predictive Maintenance für vernetzte Teilmengen-Kombinationswaagen
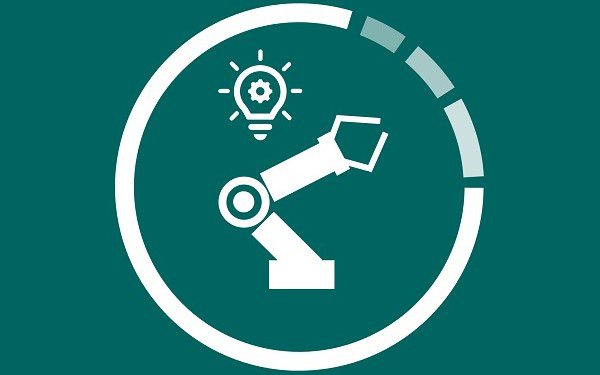
SaMoA
Automatisierte und unkomplizierte Einführung intelligenter Monitoring-Anwendung, von der benötigten Hardware bis hin zur Software.
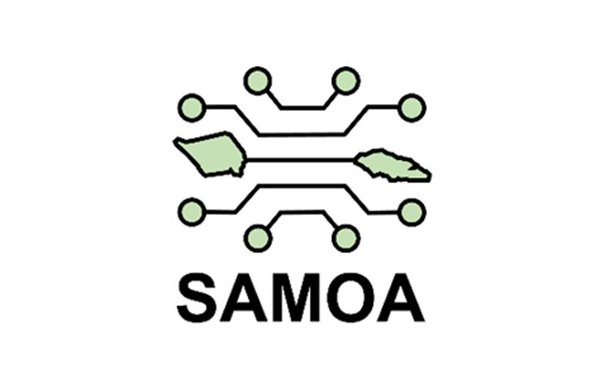
SmartCut
Smarte Lösungen für zerspanende Bearbeitungsprozesse durch den Einsatz geeigneter Sensorik und die Fusion derer Daten
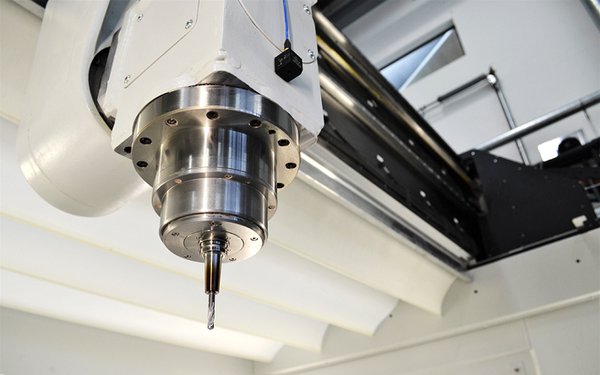