Deep 3D Athletics
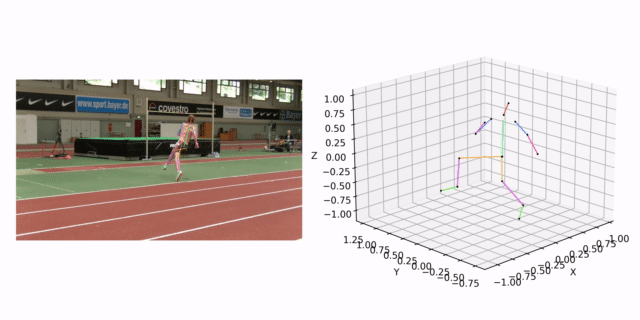
Previous projects have shown that the current state of vision-related machine learning, and espeically human pose estimation, can be integrated into applications for automated performance assessment in professional sports. The specific applicaitons included the automatic localization of athlete keypoints in video recordings as well as the derivation of key motion events. This has greatly reduced the manual annotation labor at the collaborating Olympic Training Centers (OSPs) and made their assessment service much more available.
Current evaluation is however limited to single camera footage. All motion analysis is only performed in the 2D image plane. This limits the set of kinematic parameters that can be derive from the 2D pose estimates, especially when the main motion trajectory is not perpedicular to the image plane. In this project we aim to lift the evaluation to 3D space. Current solutions for a true 3D evaluation typically require either a very expensive mutli-camera array with markerless tracking software or an enormous amount of maunal annotations. Our goal is to incorporate the latest reserach on 2D-to-3D human pose uplifting to develop an automated solution for 3D human pose estimation from calibrated camera pairs and even single monocular recordings. Our prior reserach on efficient 2D-to-3D pose uplifting with Transformers acts as the main building block. The main focus lies on efficient video processing with a high throughput and a high degree of temporal conditioning for robust and plausible 3D motion trajectories. The final goal is to deploy the developed solutions at the collaborating OSPs Hessen and Stuttgart and integrate them in their day-to-day peformance evaluaion routines for Long-, Triple- and Highjump athletes.
This joint project is funded by the Federal Institute for Sports Science (Bundesinstitut für Sportwissenschaft, BISp) based on a resolution of the German Bundestag, starting June 2022.
References:
- Moritz Einfalt, Katja Ludwig, Rainer Lienhart
Uplift and Upsample: Efficient 3D Human Pose Estimation with Uplifting Transformers
IEEE/CVF Winter Conference on Applications of Computer Vision (WACV). Waikoloa, USA, January 2023.[ arXiv][ CVF][ GitHub]
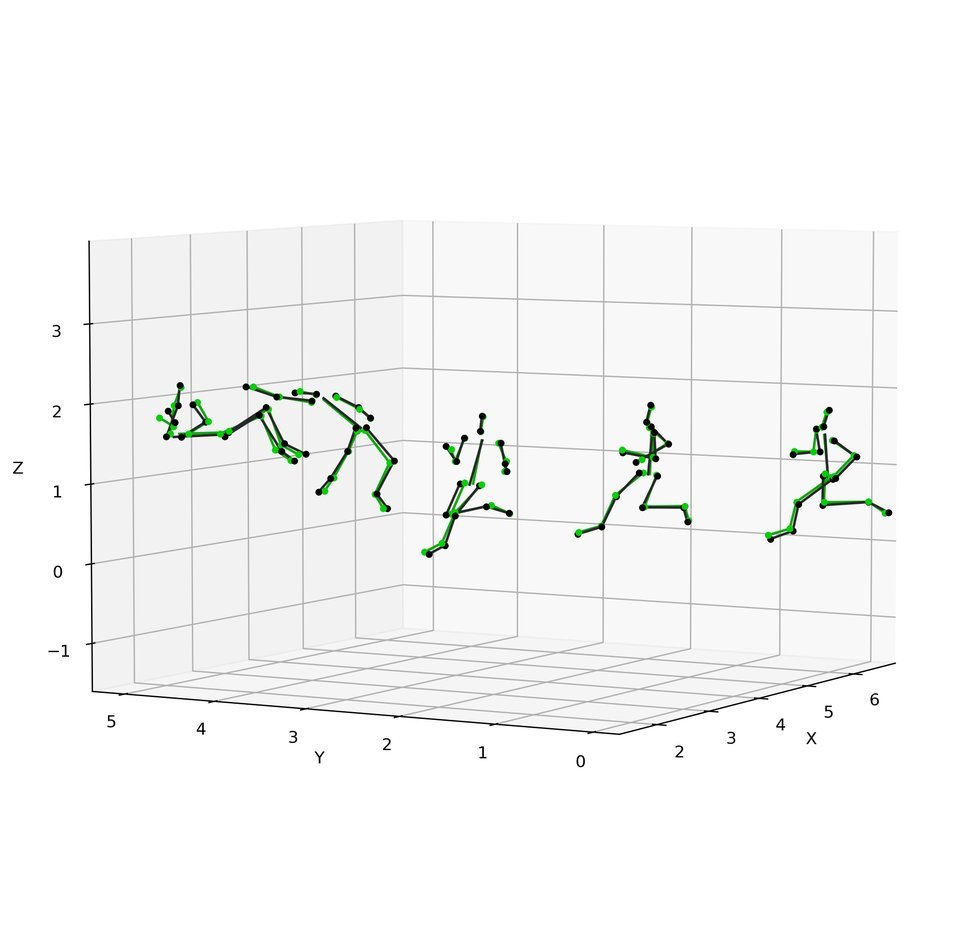
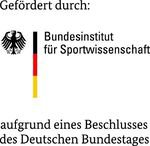