Summer School - Uncertainty, Adaptivity, and Machine Learning
Details
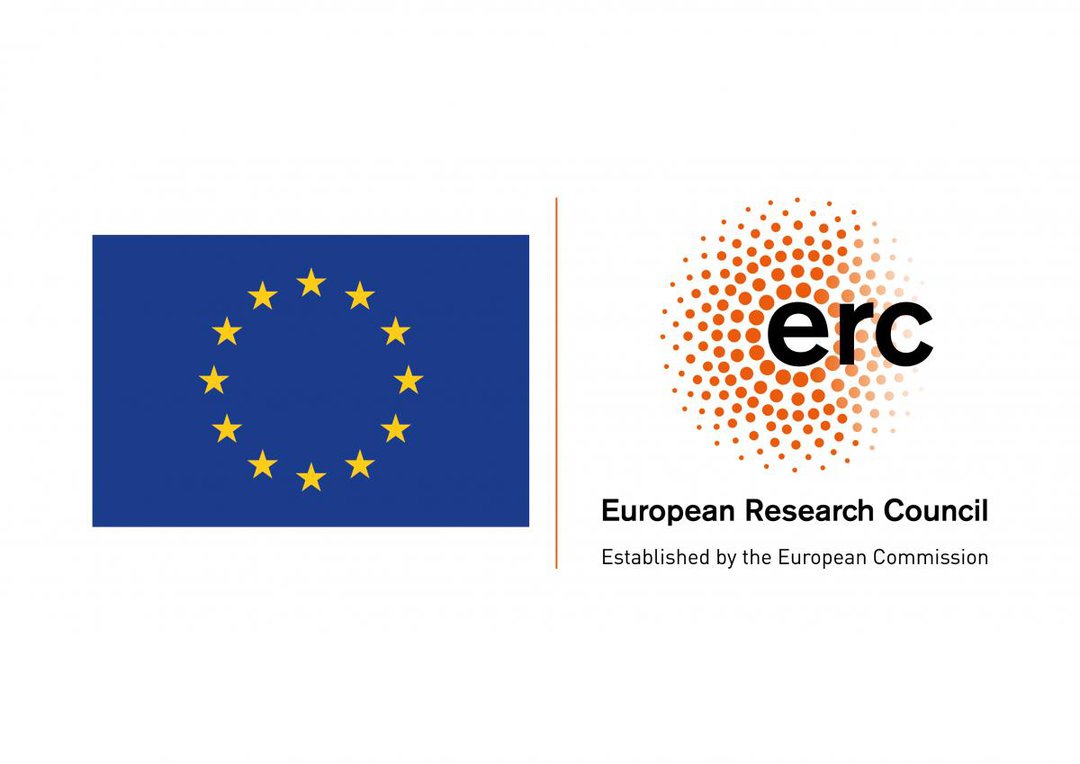
Dates: 12. - 14. September 2022
Location: Augsburg University, Institute of Mathematics, building: J, room: 1101/1102
Organizers: Philip Freese, Roland Maier (U Jena), Katrin Mang (U Hannover), Daniel Peterseim
Registration: Free and open until August 1, 2022. Please e-mail us with name, affiliation and career stage to
uam_school@math.uni-augsburg.de
Travel reimbursement is possible: If you are interested, please attach a CV and a short application text to your registration. The deadline for travel reimbursement requests is July 1, 2022.
This summer school is part of a project that has received funding from the European Research Council (ERC) under the European Union’s Horizon 2020 research and innovation programme (Grant agreement No. 865751)
Note: This event can be perfectly combined with the participation in the Chemnitz Finite Element Symposium 2022 in Herrsching from September 15 to 17, 2022.
Abstract
The summer school focuses on the numerical solution of partial differential equations (PDEs) that possibly include randomness. Modeling and discretization of random functions will be addressed, as well as Monte-Carlo-type strategies and stochastic Galerkin methods to tackle PDEs with uncertainty. Besides, the question of how to recover information about a (partially) unknown coefficient by appropriately adopting Bayesian numerical methods and making them computationally feasible will be covered. Finally, optimal adaptive discretizations for deterministic and stochastic PDEs will be discussed in the sense of obtaining the best possible results with a certain number of degrees of freedom. In this context, also machine learning techniques can be applied.
Program
Confirmed speakers:
-
Michael Feischl (Technical University of Vienna)
Optimality of Adaptive Discretizations:
The overall theme is "Optimality of Adaptive Discretizations", where optimality is understood as the best possible error with respect to a given number of degrees of freedom. This direction will be pursued for deterministic as well as for stochastic PDEs.
-
Robert Scheichl (Heidelberg University)
Numerical Methods for Bayesian Inverse Problems:
Many processes in science and engineering can be modeled via differential equations. Assuming complete knowledge of all the necessary coefficients, initial and boundary conditions, the solution of such a differential equation allows in principle to fully predict the process. Unfortunately it is rare that all coefficients are known. The inverse problem of determining the coefficients from (partial) information of the solution is significantly more complicated. A powerful way to tackle such inverse problems is to adopt a (probabilistic) Bayesian point of view. However, especially for large-scale PDE-constrained inverse problems, Bayesian numerical methods can be extremely expensive. In this short course, I will present a few novel approaches to significantly reduce this high computational cost.
-
Elisabeth Ullmann (Technical University of Munich)
Numerical Methods for Uncertainty Quantification:
In this short course we discuss the numerical solution of PDEs with random inputs. This includes models and discretizations for random functions, Monte-Carlo-type approaches and stochastic Galerkin finite element methods. The ideas are demonstrated using an elliptic PDE problem with random coefficient function.
Rough Schedule:
Monday | Tuesday | Wednesday | |
---|---|---|---|
09:00-12:00 | Lectures | Lectures | |
12:00-16:00 | Registration and Lectures | Lectures | Lectures and Closing |
16:00-17:30 | Lectures | Presentation of participants | |
18:00- | Social Event | Dinner | |
19:00- |
Detailed Schedule (updated September 05, 2022)